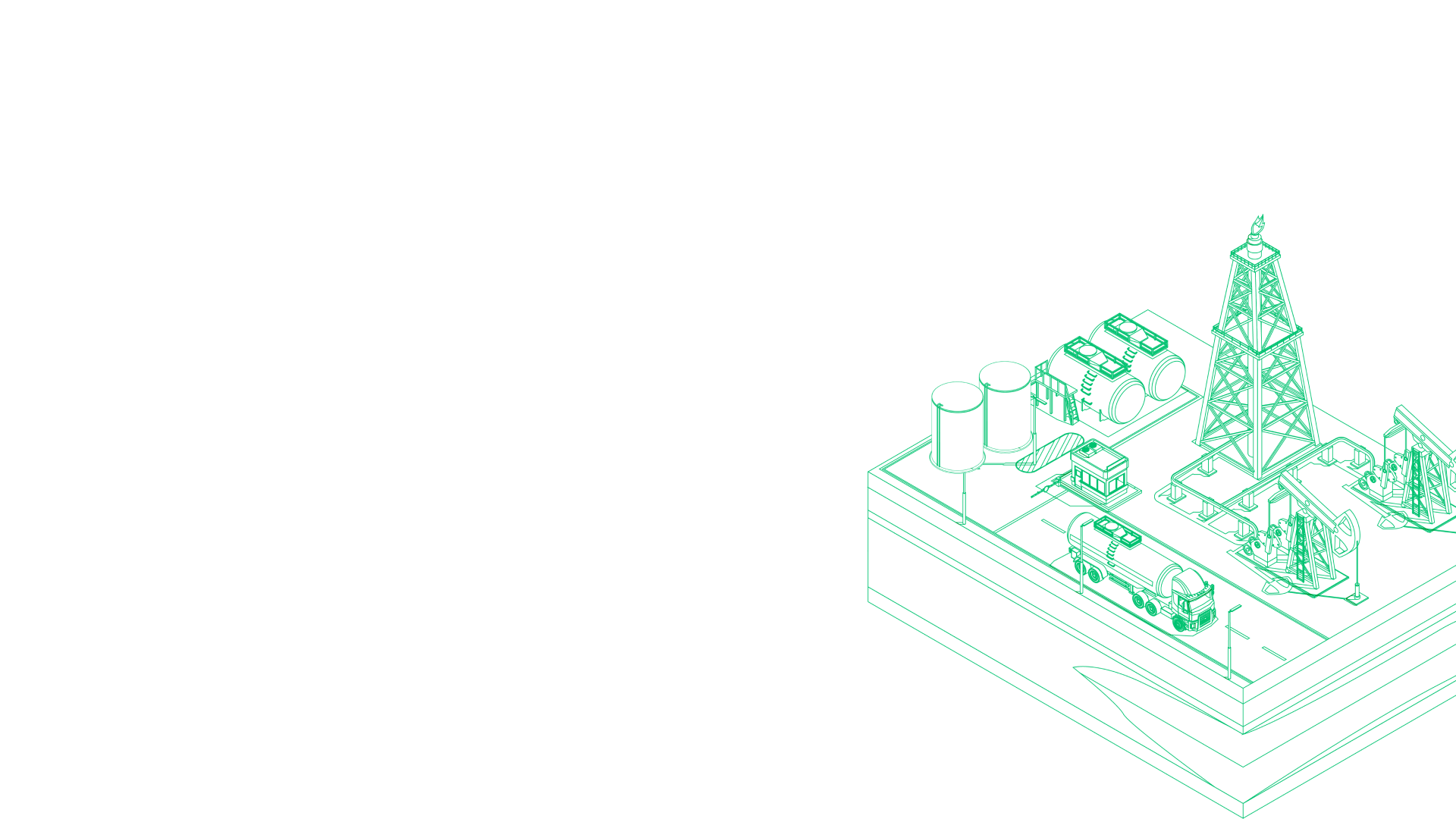
Expert Industry Insights
Get unique insights from Qube Technologies' experts to make better decisions and foster innovation.
Qube Technologies Wins Industry Innovation Award from Western Energy Alliance
Qube Technologies is proud to receive the Industry Innovation Award from Western Energy Alliance.
The award recognizes Qube’s leadership in developing real-time methane monitoring technology for oil and gas operators. The Western Energy Alliance celebrates companies and individuals making a lasting impact on the industry across the western United States.
Maas Energy Works Expands Partnership with Qube Technologies to Monitor Biogas Operations Across Multiple States
Qube Technologies, a leader in continuous emissions monitoring, today announced an expanded partnership with Maas Energy Works (MEW) to deploy Qube’s monitoring devices across more than 20 lagoon-style digesters in Texas, Idaho, and California. Following the success of an initial pilot, MEW will now implement Qube’s real-time monitoring solution across its broader network to enhance operational visibility, reduce emissions, and optimize biogas production at scale.
Qube’s Continuous Monitoring Uncovers Blocked Vent Line: Emissions Drop 80% at Colorado Wellsite
At a Colorado wellsite, Qube’s continuous monitoring system alerted operators to a sustained emission event. Qube’s data localized the emissions to the tank area and prompted a field investigation to verify the source of the anomaly. With this information, the team was able to identify the emission source and interrogate the root cause, a blocked vent line leading to the combustor.
Qube Platform Release 2.50: Smarter Alarms & Improved Emission Analytics
Platform Release 2.50 is now live! This update delivers more user alarm control and visualized insights, leading to better operational performance.
Rapid Leak Detection in the Piceance Basin: How Qube Helped Catch A Separator Leak
In Colorado’s Piceance Basin, a leading operator continues to set the bar for emissions control and operational safety. By integrating Qube Technologies’ continuous methane monitoring system, the operator aimed to strengthen its LDAR program and protect nearby homes located within 1,000 feet of its well pad.
This case study details how real-time alarms and accurate emissions source localization led to rapid action at the wellsite. Notably, catching a high-volume leak at the separator from going unnoticed over the weekend.
Certified Secure: Qube’s Continuous Methane Monitoring Platform Meets SOC 2 Type II Standards
Qube has officially passed its SOC 2 Type II audit. This achievement confirms that our controls, processes, and infrastructure consistently meet high standards for data security, availability, and confidentiality.
Smarter Sampling, Sharper Signals: How Qube Cuts Bandwidth and Power Use in Methane Monitoring
Continuous monitoring provides a clear advantage: it constantly captures emissions data 24 hours a day. But this volume of data comes at a significant cost of bandwidth and power.
How can operators maintain reliable monitoring without straining their networks or batteries? Qube solves this by combining efficient power use with intelligent sampling techniques.
Here we review the advances in sampling, data compression, and payload frequency that make this possible.
Qube Technologies Expands Partnership with Roeslein Renewables to Monitor Biogas Operations Across Multiple States
Qube Technologies, a leader in methane emissions monitoring solutions, today announced an expanded partnership with Roeslein Renewables to monitor methane emissions across their biogas operations. Building on the success of a pilot program monitoring 18 lagoons, Roeslein will deploy additional Qube devices across 100 lagoons in Missouri, Kansas, Texas, Oklahoma, and North Carolina, encompassing their entire operational footprint.
Qube’s Data Driven Approach to Optimizing Sensor Placement
Strategic sensor placement is critical for reliable, real-time methane detection. Detection depends not just on technology, but on understanding wind behavior, emission sources, and site constraints.
Qube’s deployment methodology uses historical wind data, source mapping, and MILP-based optimization to design sensor arrays that maximize detection coverage and minimize false negatives.
The Qube deployment tool turns this methodology into a practical workflow—helping operators visualize trade-offs, configure sensor layouts, and balance detection accuracy with budget.
Qube Platform Release 2.49: Improved Overview Page Filtering, New Emission Analytics
Platform Release 2.49 is now live! Save time and gain insights from improved filtering and analysis.
How Qube’s Automated Alarms Caught a Cow at the Wellsite
A Colorado operator using Qube’s continuous methane monitoring system received an automated alert about an unexpected emissions spike at a wellhead. Upon investigation, the field team discovered the source: a cow had rubbed against the equipment and opened a manual ball valve, unintentionally releasing gas. The team quickly shut the valve, restored normal readings, and installed fencing to prevent similar incidents. Thanks to Qube’s real-time alerts, the issue was resolved within an hour—without downtime or emissions escalation.
QB Energy and Qube Technologies Resolve Thief Hatch Leak Leading to 97% Emissions Reduction
“Another Win for Continuous Monitoring”
-Air Compliance Manager-QB Energy
Missed by AVO, caught by continuous monitoring. When Qube Technologies flagged elevated methane at a QB Energy tank site, field teams were able to locate and fix a leaking thief hatch—achieving a 97% emissions drop in hours. This case study shows how real-time continuous monitoring data and a fast response can keep operations running smooth.
How Qube Helped One Permian Operator Detect Offsite Emissions
Qube Technologies’ continuous emissions monitoring solutions provide operators with the tools to differentiate offsite from onsite emissions. Furthermore, our data modeling and intuitive dashboard omit those outside emissions from your facility total.
Qube Platform Release 2.48: Better Support Visibility, Smarter Data Exports, and Streamlined Alarm Resolution
Release 2.48 is now live! Enhanced fleet visibility. Smarter data exports. Streamlined alarm resolution.
Employee Spotlight: Gretchen Kern, VP of Regulatory and External Affairs at Qube
As VP of Regulatory and External Affairs, Gretchen brings her passion for environmental progress to Qube. "My favorite thing to do is advocacy in Washington, DC," she explains. Her work involves engaging with regulatory agencies, members of Congress, industry groups, and think tanks, including participation in the prestigious Harvard Methane Roundtable.
Qube Technologies Leads in Continuous Methane Monitoring: Independent Trials Confirm Best-in-Class Landfill Performance
Independent trials by FluxLab and the Environmental Research & Education Foundation (EREF) confirmed Qube Technologies as the top performer in continuous landfill methane monitoring. In two independent trials, Qube’s continuous monitoring system delivered the most accurate and resilient results—even under extreme weather conditions. With high-resolution, real-time data, Qube empowers landfill operators to better detect, quantify, and reduce emissions. The takeaway? Science-backed performance you can trust..
AER Directive 060 Updates: Streamlined Compliance with Qube’s Continuous Monitoring Solution
Explore key updates to Alberta Directive 060 simplifying alt-FEMP adoption and learn how Qube’s continuous monitoring ensures seamless compliance and efficiency.
The Business Case for Continuous Methane Monitoring in Oil & Gas Operations
Methane management has emerged as a strategic priority for oil and gas operators. As regulatory frameworks evolve and investor expectations sharpen, reliance on periodic leak detection methods is no longer sufficient.
Qube Employee Spotlight: Nolan Osiowy - Helping Customers Unlock the Power of Emissions Monitoring
Originally from Calgary, Nolan pursued a bachelor's degree in mechanical engineering at the University of Calgary. Even during his studies, Nolan was drawn to the intersection of engineering, environmental science, and energy - working summer roles at oil and gas operators in northern Alberta and BC.
Qube Platform Release 2.47: New Tools to Improve Emissions Management
We’re excited to announce Qube Dashboard Release 2.47, which introduces key upgrades to improve your emissions tracking and analysis. This release focuses on enhancing the user experience, streamlining data analysis, and helping you act faster when it matters most.